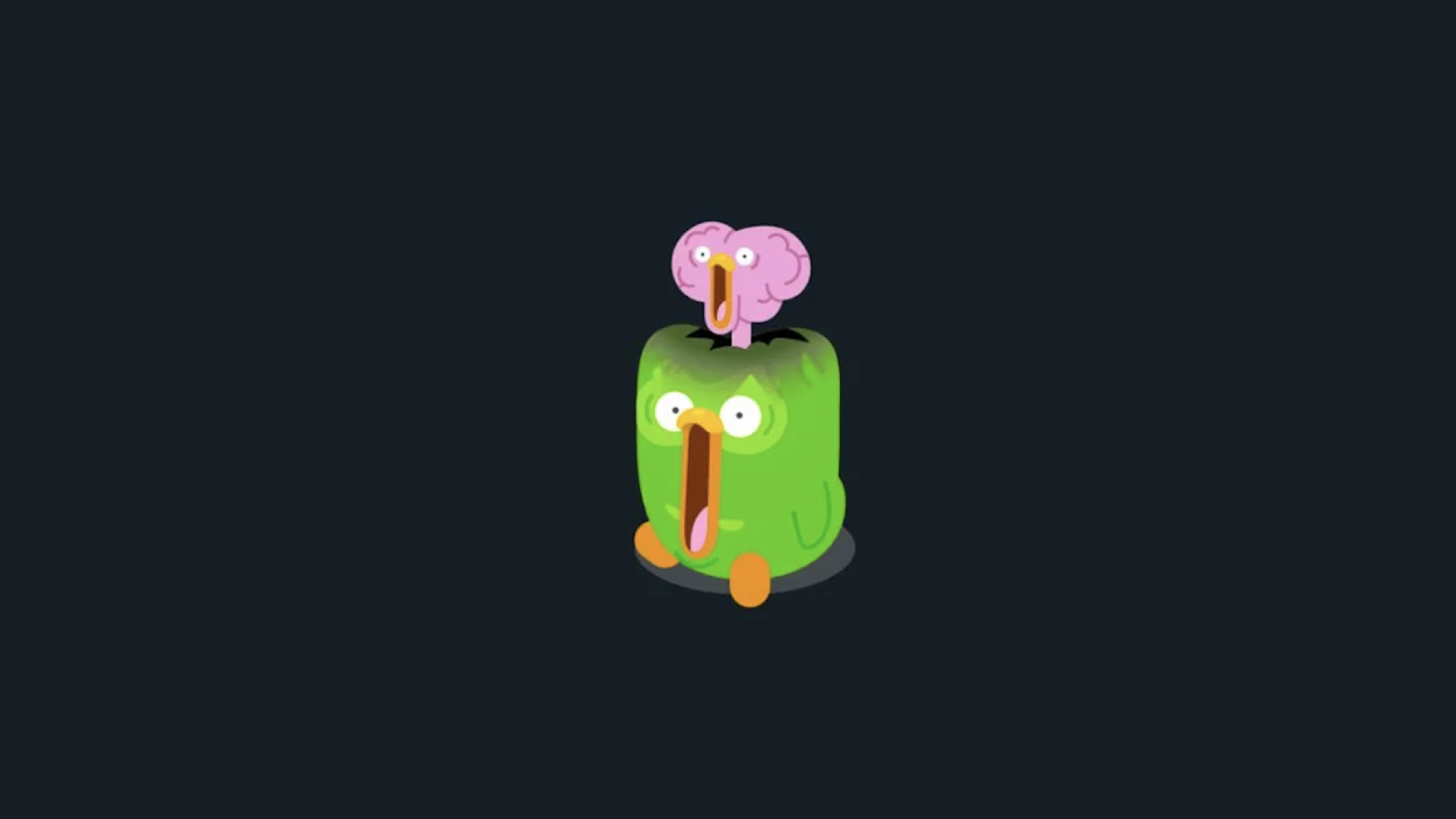
In recent months, Duolingo and Shopify have joined the growing ranks of companies announcing that they are adopting an “AI-first” approach to their business.
These announcements come at a moment when AI has taken center stage in reshaping the government, and everyone is questioning how AI will transform work, the job market, and what it all means for the economy at large.
They also mark the beginning of a new era. AI is no longer simply a tool or technology. It embodies a new corporate ideology that values speed, efficiency, and growth, with little (at least explicit) thought given to the value it’s creating for users or its impact on society.
This raises the question: is the AI-first mentality put forward by companies like Shopify and Duolingo just the next stage of what the tech writer Cory Doctrow has termed “enshittification“? In other words, is AI-first about building better products and stronger businesses, or simply about boosting short-term, bottom-line metrics at the expense of long-term value
What is AI-first?
On the surface, AI-first can sound like other technology-led shifts we’ve seen before: mobile-first, cloud-first, digital-first, etc. But unlike these previous shifts, AI-first is lacking the key driver that made these movements so successful: users.
While Shopify rode the wave of its cloud-first approach early on and the Duolingo memo references the importance of a mobile-first strategy in its own early success, AI-first is fundamentally different. Unlike AI-first, these shifts weren’t really about technology; they were about adapting to changing user or customer needs.
Mobile-first, for example, wasn’t about screens. It was about designing for how people actually engage with technology, on the go, in real-time, on smaller devices. It forced simplicity and responsiveness in UX.
Similarily, cloud-first wasn’t about servers, but about making data and tools accessible, enabling remote work, real-time collaboration, and global scalability.
Both were technology shifts in the service of users. They solved clear problems and reshaped how businesses delivered value.
Is AI-first the new “move fast and break things”?
But AI-first feels different. Less like a strategy and more like a slogan, which in some ways it recalls another era-defining tech mantra: “Move fast and break things.”
What was once used to justify disruption in the name of progress (sometimes with dire consequences we are only just beginning to realize) now seems to be resurfacing, only this time, it’s powered by AI and wrapped in corporate memos. The message is the same: speed over stability, outputs over outcomes, action over accountability.
As laid out in the memos of Duolingo and Shopify, AI-first isn’t about meeting customers where they are (mobile-first) or improving customer experience through technology (cloud-first); it’s about who does the work, how decisions get made, and what kinds of work are valued. In short, there are no explicit human needs driving this shift.
Taken to its logical conclusion, the AI-first companies envisioned in these memos start to sound dangerously close to the “enshittogenic environment” Cory Doctrow blames for “ruining the internet:”
“An enshittogenic environment meant that individuals within companies who embraced plans to worsen things to juice profits were promoted, displacing workers and managers who felt an ethical or professional obligation to make good and useful things. Top tech bosses — the C-suite — went from being surrounded by “adult supervision” who checked their worst impulses with dire warnings about competition, government punishments, or worker revolt to being encysted in a casing of enthusiastic enshittifiers who competed to see who could come up with the most outrageously enshittificatory gambits.”
AI isn’t pixie dust.
To understand why AI-first initiatives often fall flat, it’s worth looking back to 2020. Long before ChatGPT and the latest wave of AI hype, Google’s Will Grannis issued a now-prescient warning:
“AI isn’t pixie dust. It’s not as simple as ‘AI is the magic answer!’ You can’t just sprinkle it on and expect success.”
At the time, Google had already declared itself an AI-first company. But even then, Grannis cautioned against starting with AI. Instead, he described AI as a way to optimize or unblock what’s already working, not replace it wholesale. His advice was to ask a simple, human-centered question:
“Have I already realized all of the value that analytics and subject matter experts have to give? “
AI has come a long way since 2020, and while Grannis’s question may seem quaint today, it raises other important questions that too easily get lost in the AI bluster:
How can we use AI to augment, expand, and scale what we are already doing, rather than just assuming that AI will be better at any given task than a human is?
In other words, how can we use AI to solve real problems and make things better?
Not everything is a nail.
This is the work. Figuring out what’s not working and making it better. Instead of using AI as “a hammer when you’re trying to attach a bolt,” looking at customer pain points, operational friction, or market gaps, and saying:
We’ve really been having a hard time with X. For years, we’ve had a goal of getting better at it, but we just haven’t gotten there, or think we could do more — is this something AI could help us with?
Which also requires being honest about the tradeoffs of using AI:
- Will it reduce quality, trust, or user experience?
- Will it shift work to customers in ways that create friction?
- Will it undermine our employees’ expertise, autonomy, or engagement?
- Are we willing to accept accountability for its performance?
Unfortunately, that’s not the conversation most AI-first companies are starting. Instead, they sound more like this:
We’d like to cut costs and move faster. Go figure out how AI can do that.
The problem isn’t defined. The value isn’t clear. And the burden is shifted from leadership to employees, without the strategy, support, or measurement needed for them to succeed.
Who is AI-first really for?
As publicly traded companies whose stock prices surged after these memos were published, it seems reasonable to conclude that customer pain points and user needs aren’t what’s driving the AI-first shift at Duolingo and Shopify.
While the Shopify memo nods to its mission of using AI to empower entrepreneurs, reducing costs (ie, using AI to slow down hiring), driving efficiencies, and improving productivity are the stated aims of its AI-first shift. These are things that benefit shareholders and executives, with little benefit (and possibly a high cost) to customers and employees.
Employees are being told to “figure out” how to use AI in their work, not as an invitation to innovate or drive organizations forward as a whole, but as a mandate to stay relevant. It’s “empowerment” with a winking threat.
That’s one way to approach the AI question — but even so, the ROI is far from guaranteed. Without clear metrics, targeted use cases, and a deep understanding of user needs, what looks like efficiency today can become a vulnerability tomorrow.
Look no further than what is currently happening at Klarna, which, after its own “AI-first proclamation,” is now hiring back customer service reps that it hastily (and disastrously) replaced with AI.
In Shopify’s recent memo, posted on X, CEO Tobias Lütke promises a “10X” future, where AI becomes a productivity multiplier. But it never says what is being multiplied or for whom. Is it customer value? Business outcomes? Or just cost-cutting?
And even the supposed productivity gains are hard to pin down. What are they based on? More tasks completed? More value created? Better customer outcomes? Would the same investment in other types of training have delivered a more lasting impact? No one seems to know because that’s not how these decisions are being made.
AI experiments in search of a problem.
Across industries, companies are launching pilots, proofs of concept (POCs), and AI-powered features at breakneck pace. In some ways, it’s reminiscent of the Google 20% Time policy that led to lucrative innovations like Gmail and Google AdSense (a comparison I’m sure CEOs would welcome).
But today’s employees aren’t being encouraged to follow passion projects; they’re being ordered to do something, anything, with AI. One executive we spoke with recently called this the POC petting zoo: a lot of shiny objects, but no meaningful outcomes.
Figma’s 2025 AI report reinforced this gap. 9% of AI builders say revenue growth is the primary goal of their projects. The vast majority? They’re “experimenting with AI.” Experimenting but without a clear problem, without customer insight, and without metrics for success.
It’s tech-first thinking, not value-first thinking. And it’s a recipe for wasted investment and missed (sometimes un-sexy, non–AI) opportunities.
The pressure to do something is understandable. It’s coming from boards, investors, and internal leadership. But it’s leading to a kind of reactive churn, one that, while it may benefit shareholders and executives in the short term, risks leaving both users and employees empty-handed.
From AI-first back to first principles.
At Cake & Arrow, we’ve seen firsthand how the rush to adopt technology can outpace the clarity needed to use it effectively. This is especially true in insurance, where trust, empathy, and nuance matter.
The real opportunity isn’t in just being first. It’s in being thoughtful and strategic about how you use AI. Clear about what problem AI is solving. Clear about how it improves the experience for customers, employees, or the business. Clear that the task we’re assigning is one AI is uniquely qualified to do. Clear about what success looks like — and how it is measured.
AI is really exciting. It is full of potential. But it isn’t inherently valuable on its own. Like any technology, it’s valuable when it makes something better, simpler, more useful, more human. When it doesn’t, it’s not just wasted effort. It can erode trust, damage the product, and leave companies with burnt-out teams, disengaged users, and a weakened brand.
Transformational AI doesn’t start with a press release, a pilot, or a post on X or LinkedIn. It starts with a problem you want to solve. It starts with people.
It’s time to redefine what ‘AI-first’ means — not as a race to adopt new tools, but a strategy rooted in solving real problems. Here’s how we think mindsets around AI-first might shift to better serve businesses, employees, and the people and the products they use:
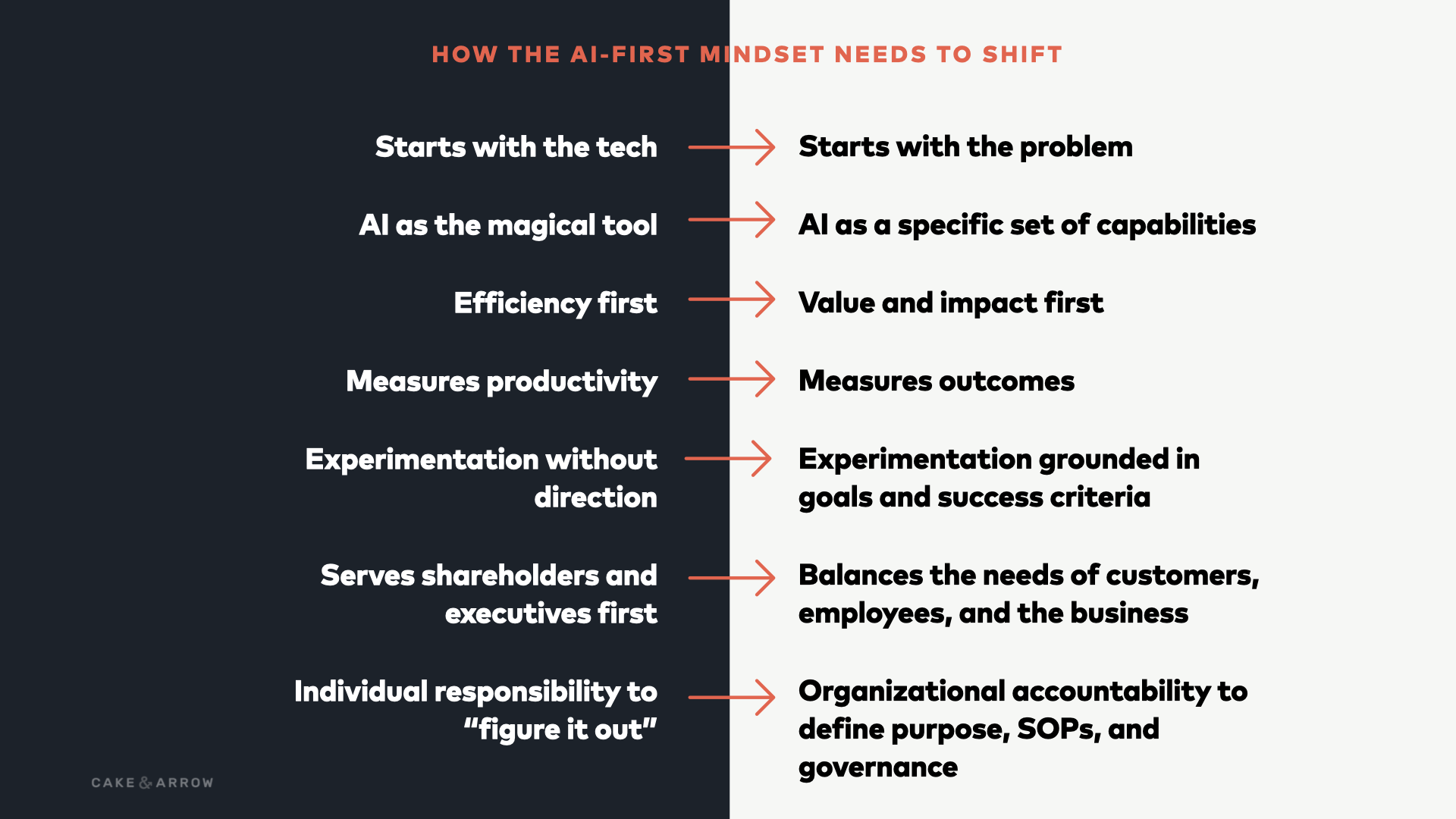